confounded(Confounded Understanding the Complexity of Confounding Variables)
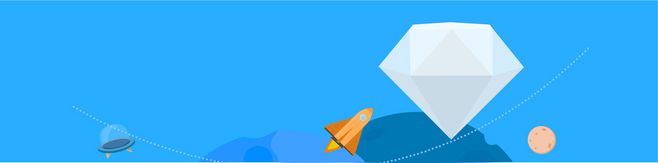
Confounded: Understanding the Complexity of Confounding Variables
Introduction
Confounding variables play a significant role in every research study, often leading to inaccurate or misleading results. These variables can obscure the true relationship between the independent and dependent variables, making it difficult to establish causal links. Understanding confounding variables and their effects is crucial for researchers, as it helps ensure the validity and reliability of the study findings. In this article, we will explore the concept of confounding variables, discuss their impact on research, and highlight strategies to mitigate their effects.
The Nature of Confounding Variables
Confounding variables, also known as extraneous variables, are factors that influence both the independent and dependent variables in a research study. They are often unseen and unexpected and can distort the true relationship between variables. To comprehend the impact of confounding variables, consider a study on the relationship between coffee consumption and the risk of heart disease. If age is not controlled for as a confounding variable, it may lead to a false association between the two variables. Older individuals are more likely to consume coffee and also have a higher risk of heart disease, introducing a confounding effect. Therefore, age acts as a confounding variable, creating a spurious correlation.
Effects of Confounding Variables
Confounding variables can have detrimental effects on the validity of research findings. Without proper control or consideration of these variables, researchers run the risk of drawing incorrect conclusions. Confounding can lead to both overestimation and underestimation of the effect of the independent variable on the dependent variable.Overestimation occurs when a confounding variable amplifies the perceived effect of the independent variable. Continuing with the previous example, if individuals who consume more coffee also tend to engage in more physical exercise, the association between coffee consumption and heart disease risk may be exaggerated. This overestimation can have significant implications, leading to misguided public health recommendations or ineffective intervention strategies.
Conversely, underestimation happens when a confounding variable masks the true effect of the independent variable. For example, if individuals with a family history of heart disease are more likely to avoid coffee consumption, failing to account for this family history as a confounder might underestimate the potentially protective effect of coffee on heart health. As a result, important relationships and correlations may be overlooked or dismissed.
Addressing Confounding Variables
Recognizing and controlling for confounding variables is essential to ensure the internal validity of a study. Researchers utilize various strategies to mitigate the effects of these variables.One common approach is randomization. Random assignment of participants to different groups or conditions helps distribute confounding variables evenly and minimizes the likelihood of bias. By ensuring that confounders are approximately equally distributed across all groups, researchers can confidently attribute any observed effects to the independent variable.
Another strategy is matching. This technique involves selecting participants or creating groups that are similar in terms of potential confounding variables. By matching individuals who share similar characteristics, researchers can account for potential confounders and establish a clearer relationship between the independent and dependent variables.
Additionally, statistical techniques such as stratification and regression analysis can be employed to adjust for confounding variables. These methods allow researchers to control for the effects of specific variables by statistically accounting for them in the analysis. Through careful consideration and utilization of appropriate statistical models, researchers can accurately identify and isolate the true effects of the independent variable on the dependent variable.